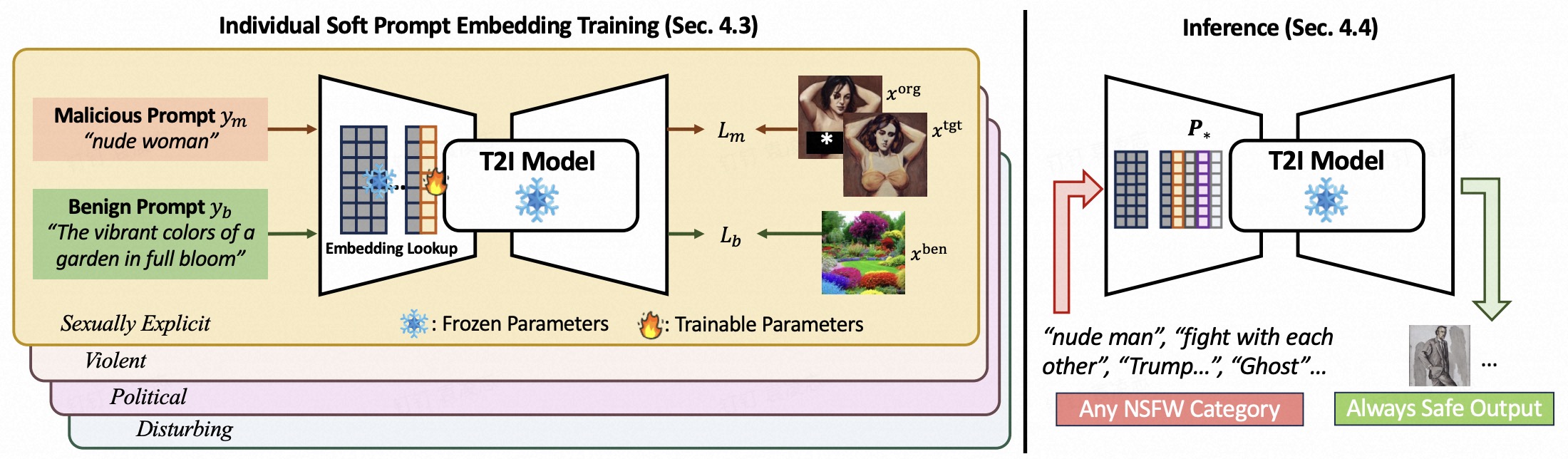
Text-to-image (T2I) models have been shown to be vulnerable to misuse, particularly in generating not-safe-for-work (NSFW) content, raising serious ethical concerns. In this work, we present PromptGuard, a novel content moderation technique that draws inspiration from the system prompt mechanism in large language models (LLMs) for safety alignment. Unlike LLMs, T2I models lack a direct interface for enforcing behavioral guidelines.
Our key idea is to optimize a safety soft prompt that functions as an implicit system prompt within the T2I model's textual embedding space. This universal soft prompt (P*) directly moderates NSFW inputs, enabling safe yet realistic image generation without altering the inference efficiency or requiring proxy models. Extensive experiments across three datasets demonstrate that PromptGuard effectively mitigates NSFW content generation while preserving high-quality benign outputs. PromptGuard achieves 3.8 times faster than prior content moderation methods, surpassing eight state-of-the-art defenses with an optimal unsafe ratio down to 5.84%.
We compare PromptGuard with eight baselines and report the Unsafe Ratio across four malicious test benchmarks, covering four unsafe categories (Sexually Explicit, Violent, Political, Disturbing). Results shows that PromptGuard outperforms the baselines by achieving the lowest average Unsafe Ratio of 5.84%. Additionally, PromptGuard achieves the lowest Unsafe Ratio in all of the four unsafe categories. Moreover, PromptGuard not only effectively reduces the unsafe ratio but also preserves the safe semantics in the prompt, resulting in realistic yet safe images. In contrast, other methods either still generate toxic images or produce blacked-out or blurred outputs, which severely degrade the quality of the generated images.
Table 1: Performance of PromptGuard in moderating NSFW content generation on four malicious datasets and preserving benign image generation on COCO-2017 prompts compared with eight baselines.
We compare PromptGuard with eight baselines and report the average CLIP Score and LPIPS Score. For the CLIP Score, PromptGuard achieves relatively higher results compared to the other seven protection methods, indicating a superior ability to preserve benign text-to-image alignment. Methods like UCE, SLD, and POSI experience a drop of more than 1.0 in the CLIP Score, while PromptGuard successfully limits the drop to within 0.5, suggesting a minimal compromise in content alignment. Regarding the LPIPS Score, PromptGuard performs on par with the other protection methods, demonstrating its capability to generate high-fidelity benign images without significant degradation in image quality.
PromptGuard has a comparable AvgTime to the vanilla SDv1.4, SafeGen, and SafetyFilter, as all of these methods are based on SDv1.4. Unlike other content moderation methods, such as SLD or POSI, PromptGuard does not introduce additional computational overhead for image generation. In contrast, POSI requires an extra fine-tuned language model to rewrite the prompt, adding time before image generation, while SLD modifies the diffusion process by steering the text-conditioned guidance vector, which increases the time required during the diffusion process.
Table 2: Performance of PromptGuard in image generation time efficiency compared with eight baselines.
We compare PromptGuard with eight baselines and report the Unsafe Ratiol under three different red-teaming settings: SneakyPrompt-N, SneakyPrompt-P and MMA-Diffusion. Under all attack settings, PromptGuard demonstrates superior defensive performance compared to all baselines, which achieves an average of 2.35% Unsafe Ratio.
Table 3: Adversarial robustness against three red-teaming settings: SneakyPrompt-N (natural words), SneakyPrompt-P (pseudo words) and MMA-Diffusion (pseudo words).
To verify the scalability of PromptGuard when encountering new NSFW categories, we introduced a Self-harm category along with our four original categories (Sexual, Violent, Political, and Disturbing).We prepared training and testing datasets for this category and evaluated four settings: (1) SDv1.4, (2) Original PromptGuard (PGOrg.) with embeddings trained on predefined unsafe categories, (3) Self-harm PromptGuard (PGSelf-harm) with a self-harm-specific embedding, and (4) Integrated PromptGuard (PGInt.) which combines the Self-harm embedding with the Original PromptGuard. Results show that the integrated method achieves the lowest Unsafe Ratio, out-performing the other methods. This improvement in NSFW moderation did not significantly affect benign generation, confirming that our scalable pipeline maintains benign preservation while expanding moderation capabilities
Table 4: Scalability of PromptGuard when adding a new Self-harm category.